[Update August 2018] Unfortunately Spot the Odd Song Out is offline as it needs some updates to the latest web developments. If you are interested contributing to the development of a modern music-based Games-With-A-Purpose platform, please contact Daniel directly at [daniel(dot)wolff(dot)2(at)city.ac.uk]. A video of the game can be seen below.
Spot the Odd Song Out is a multi player music discovery game: Listen to popular songs and get the best high score to prove you are an expert in music similarity. You can join and leave a speedy multi player game at any time you want. Get to the higher levels in order to choose your favourite music genre, or a fancy avatar!
We would be very happy if you try the game on the web and contribute to our research!
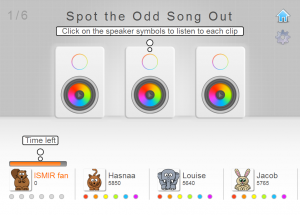
Spot the Odd Song Out is part of a research project at City University London in collaboration with Guillaume Bellec at ENSTA Paristech. We use gaming data to understand how players judge music similarity as well as to explore perception of tempo and rhythm. In particular, we are interested in the way people compare music, and how that relates to their musical and cultural background.
Music Similarity
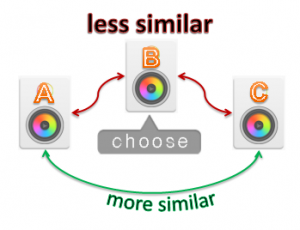
In the similarity part of the game, the team of players is presented three songs, and asked to individually choose the song which is the least similar to the remaining two (the “odd song out”). Players are awarded points if they agree on the odd song out. This data has been used by researchers to build general models for music similarity, predicting more than 70% of the users similarity input. Our experiments on the MagnaTagATune dataset which contains such similarity data are published here and here. If a user selects song B out of songs A,B,C, then songs (A and C) are expected to be more similar than (B and C) or (A and B).
Additionally to the data collected in MagnaTagATune, as we aim to measure influence of personal attributes and cultural context, the similarity statements are enriched with anonymised user attributes by Spot the Odd Song Out, linking the similarity data to age group, location, gender, or expanded geographical reach of the user. Such data allows for ethnographic and culture-aware studies of how music is perceived in different locations and cultural subgroups.
This in turn allows for more specific music similarity models which could be used for personalised music recommendation (you might know this from Last.fm, 8tracks, Spotify…) , taking into account the users’ cultural attributes such as knowledge of music genres when recommending music.
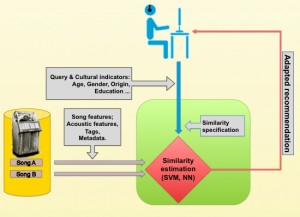
Further publications on our research on similarity are listed below.
References:
Wolff, D. (2014). Spot the Odd Song Out: Similarity Model Adaptation and Analysis using Relative Human Ratings. PhD Thesis, City University London, Aug. 2014.
Wolff, D., Weyde, T., MacFarlane A. (2013). An online game for collecting music similarity data. Talk at DMRN+8, Queen Mary University, London 2013
Wolff, D., Weyde, T. (2013). Learning music similarity from relative user ratings. In: Springer Information Retrieval, 2013. The final publication is available at springer.com
Bellec, G., Friberg, A., Wolff, D., Elowsson, A., and Weyde, T. (2013). A social network integrated game experiment to relate tapping to speed perception and explore rhythm reproduction. In: Sound and Music Computing Conference, pp. 19-26, Aug. 2013.
Wolff D., Stober, S., Nürnberger, A., Weyde, T. (2012). A Systematic Comparison of Music Similarity Adaptation Approaches. In: Proceedings of the 13th International Society for Music Information Conference. Porto, Portugal, October 2012.
Wolff D., Weyde, T. (2012). Adapting Similarity on the MagnaTagATune Database: Effects of Model and Feature Choices. In: Proceedings of 4th International Workshop on Advances in Music Information Research. Lyon, France, April 2012.
Wolff D., Weyde, T. (2011). Adapting Metrics for Music Similarity Using Comparative Ratings. In: Proceedings of the 12th International Society for Music Information Retrieval Conference. Miami, Florida, USA, October 2011.
Wolff D., Weyde, T. (2011). Combining Sources of Description for Approximating Music Similarity Ratings. In: 9th International Workshop on Adaptive Multimedia Retrieval, pp. 114-124, July 2011.